These days, big and growing enterprises are more concerned about their data and control. Technologists and entrepreneurs are scrambling to create new methods to acquire, handle, and monetize data. Of course, we all understand the importance of data. But how is this to be accomplished? This blog attempts to explain how outsourcing data mining services work for businesses, why it is important to comprehend its power, and why you should care about it.
It is important because it is critical to comprehend the power of this technique when considering how our data is used. Putting another way, data mining process allows big and growing enterprises to leverage the data you contribute to disclose more than you may expect.
Data Mining As A Process
Companies can utilize the information you submit for data mining to learn more about you than you would imagine. To most of us, the data mining process is collecting a large amount of data, having quant wizards work their arcane magic, and then knowing all of this incredible information. But how will this accomplish? What kind of information may they have?
The truth is that, while the technical working of the data mining process is reasonably tricky (they are a black box unless you are a trained statistician or computer scientist), the applications and possibilities of these approaches are quite understandable and intuitive.
For the most part, data mining services show us about very vast and complicated data sets, the kind of information that would be obvious about modest and straightforward things. For example, it can display our categories and then arrange items into predetermined categories to notify us that “one of these things is not like the other.” But what is straightforward with five data points isn’t so simple with five billion. And there is always more info nowadays. We collect considerably more than we can process. Almost every transaction or interaction generates a data signature that is captured and stored someplace. Digitalization and ubiquitous computing have made it increasingly right in our lives, keeping away from our computers. The sheer volume of data has far outstripped our ability to make sense of it.
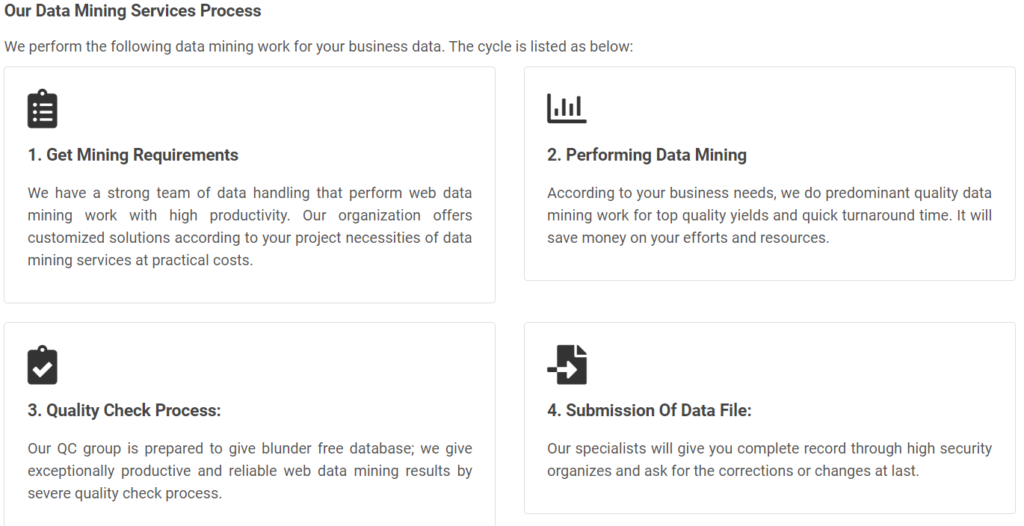
How Data Mining Helps Your Business?
Patterns are typically too faint at these scales, and linkages are too complicated or multi-dimensional to detect merely by looking at the data. Data mining helps in automating this process, allowing us to find interpretable patterns. The two main methods for extracting information from data are description and prediction, and it is not easy to know what the data reflects at the scale we are talking about.
Outsourcing data mining services allows us to simplify and summaries data to comprehend and then infer information about individual situations based on the patterns we have discovered. Of course, particular data mining applications are constrained by the amount of data and computing power available and are adapted to meet unique demands and objectives. There are, however, a few different forms of pattern detection that are routinely utilized.
These examples show what outsourcing data mining services can help to accomplish.
Anomaly Detection
With extensive data collection, it is conceivable to understand how data in a typical scenario looks. Statistics may be use to see whether anything is out of the ordinary. For example, the IRS may create a model of expected tax returns and then utilize anomaly detection to find individual returns that depart from this for inspection and audit.
Detecting Clusters
Recognizing unique clusters or sub-categories within the data is one sort of pattern recognition that is exceptionally beneficial. An analyst would have to look at the data and choose a set of categories that they feel represent the critical distinctions between apparent groupings in the data if they did not use the data mining process. It would put you in danger of overlooking essential categories. Instead, it is feasible to let the data decide the groupings via outsourcing data mining services. It is an example of a difficult-to-understand black-box algorithm. However, in a simple example involving purchasing behavior, we might suppose that the purchase habits of various hobbyists would be rather varied: gardeners, fishers, and model aeroplane enthusiasts would all be pretty different. Machine learning algorithms can identify all subgroups within a dataset that are significantly different from one another.
Learning Through Association
The Amazon recommendation system is based on association learning, which is a sort of data mining. Customers who purchased a cocktail shaker and a cocktail recipe book, for example, may also buy martini glasses. These kinds of discoveries are frequently used to target discounts and promotions, as well as advertising. Similarly, Netflix movie suggestions are based on this type of data mining (although a more advanced one).
Classification
Outsourcing Data mining services may help to categorize new instances into predetermined categories if an existing structure is already recognized. Algorithms can find permanent systemic distinctions between objects in each group and apply these rules to new classification issues after learning from an extensive range of previously classified instances. For example, large collections of spam-identified emails have enabled spam filters to detect changes in word use between genuine and spam communications, then categorize incoming messages according to these criteria with high accuracy.
Regression
Data mining process help to build prediction models based on a variety of factors using regression. For example, Facebook may be interested in forecasting a user’s future participation based on previous activity. Factors such as the quantity of personal information provided, the number of photographs tagged, started or accepted friend invitations, comments, likes, and so on might all be incorporated in such a model. As Facebook evaluates how predictions differ from actual behavior, this model might be fine-tuned over time to include or weight items differently. These findings might ultimately inform the design to promote more of the behaviors that appear to lead to increased engagement over time.
Conclusion
The descriptive data mining patterns and structures are frequently used to forecast other features of the data. Amazon is a good illustration of how descriptive data may be utilized to predict future outcomes. For example, the (hypothetical) link between cocktail shaker and martini glass purchases and many other such relationships may be utilized as a part of a model forecasting the chance that a particular user would make a specific purchase. This model might match these relationships to a user’s purchase history and indicate which goods they are most likely to buy. Amazon may then target adverts to that person depending on what they’re most likely to purchase.
In this sense, outsourcing data mining services may provide you with a lot of inference capability. For example, suppose an algorithm can accurately classify a case into a known category with little data. In that case, it may then predict a wide variety of other information about that instance using the attributes of all the other issues in that category. This may appear dry, but that is how most successful Internet businesses acquire money and gain authority.